The Voice(s) of POTUS: Using the NRC Emotion Lexicon to Predict Topical Prevalence in Donald Trump's Tweets
It's tempting to call President Donald J. Trump the "Tweeter-in-Chief," and this is true for a rather logical reason: the man tweets A LOT. I started compiling a list of the President's tweets -- both from his personal account (@realDonaldTrump) and from his official POTUS account (@POTUS) -- beginning February 16. From that date until now, Trump has tweeted more than 850 times via his personal and POTUS accounts combined.
As the below figure shows, aside from a spike in @POTUS tweets during a joint address given by Trump to Congress, Trump's Twitter activity has generally remained steady, and neither his official POTUS account nor his personal account appears to be, in general, more active.
However, similar levels of activity do not necessarily guarantee similar degrees influence. Since February 16, both Trump's personal account and the official POTUS account have seen an increase in followers; however, the former has substantially outpaced the latter in terms of both growth and total number of Twitter followers.
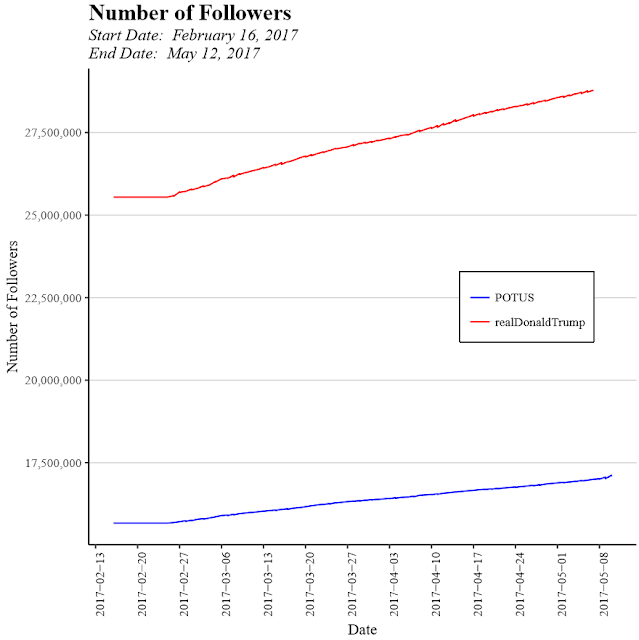
Nevertheless, millions follow both accounts, which makes each a potentially important platform for President Trump. However, an overview of both reveals clear differences in the voice and purpose of each.
To better understand these differences, I applied methods of quantitative text analysis to leverage measurable comparative insights from the composition and tone of Trump's personal and official POTUS accounts. In particular, to obtain a measure of sentiment and emotion for each Tweet in my dataset I utilized a crowdsourced dictionary of words that are coded as either positive or negative and are associated with one of eight emotions (anger, fear, joy, trust, sadness, disgust, surprise, and anticipation) [for more details, see the NRC Emotion Lexicon website]. I then used the positive and negative sentiment scores in particular to estimate sentiment valence (i.e., positive score - negative score = sentiment valence).
In addition to displaying a basic summary of results from the sentiment analysis, I additionally leverage sentiment valence as a covariate, in conjunction with Twitter account identity, in the estimation of topical prevalence via a structural topic model (STM), which applies an iterated expectation-maximization algorithm to the estimation of k user-determined topics.
As the results below show, similarities and differences between Trump's personal account and the official POTUS account are present, and the nature of these similarities and differences is relevant to an overall understanding of the impact the President's bifurcated social media presence.
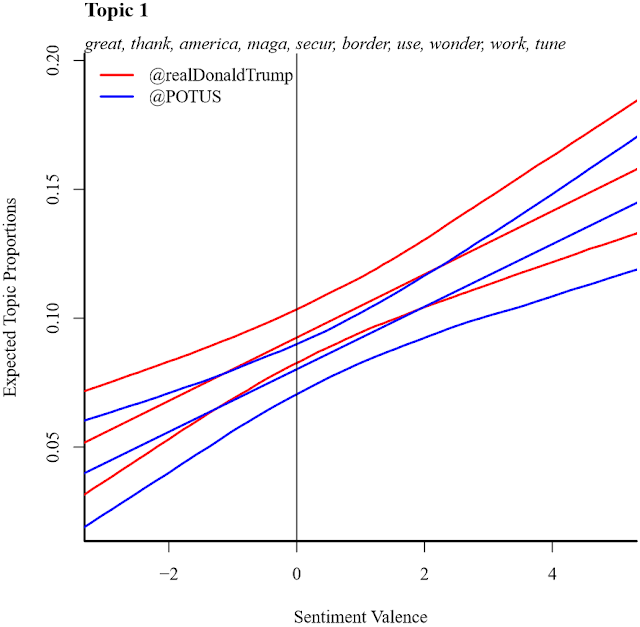
________________________________________________
See this project in my GitHub repository here.
As the below figure shows, aside from a spike in @POTUS tweets during a joint address given by Trump to Congress, Trump's Twitter activity has generally remained steady, and neither his official POTUS account nor his personal account appears to be, in general, more active.
However, similar levels of activity do not necessarily guarantee similar degrees influence. Since February 16, both Trump's personal account and the official POTUS account have seen an increase in followers; however, the former has substantially outpaced the latter in terms of both growth and total number of Twitter followers.
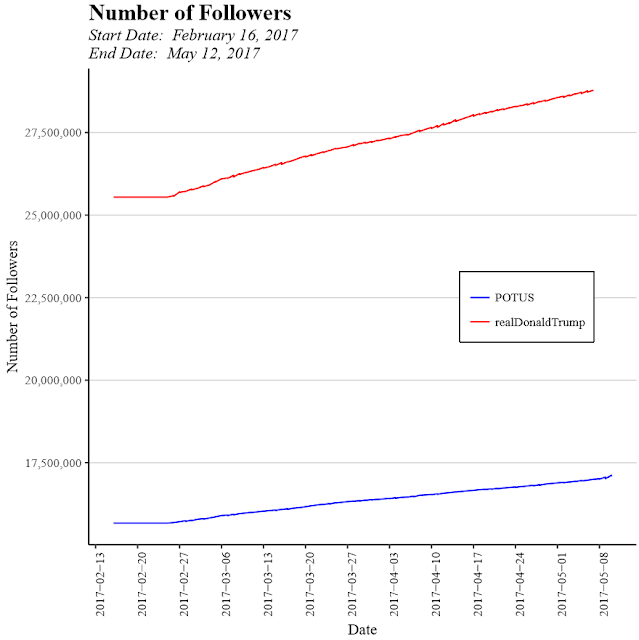
Nevertheless, millions follow both accounts, which makes each a potentially important platform for President Trump. However, an overview of both reveals clear differences in the voice and purpose of each.
To better understand these differences, I applied methods of quantitative text analysis to leverage measurable comparative insights from the composition and tone of Trump's personal and official POTUS accounts. In particular, to obtain a measure of sentiment and emotion for each Tweet in my dataset I utilized a crowdsourced dictionary of words that are coded as either positive or negative and are associated with one of eight emotions (anger, fear, joy, trust, sadness, disgust, surprise, and anticipation) [for more details, see the NRC Emotion Lexicon website]. I then used the positive and negative sentiment scores in particular to estimate sentiment valence (i.e., positive score - negative score = sentiment valence).
In addition to displaying a basic summary of results from the sentiment analysis, I additionally leverage sentiment valence as a covariate, in conjunction with Twitter account identity, in the estimation of topical prevalence via a structural topic model (STM), which applies an iterated expectation-maximization algorithm to the estimation of k user-determined topics.
As the results below show, similarities and differences between Trump's personal account and the official POTUS account are present, and the nature of these similarities and differences is relevant to an overall understanding of the impact the President's bifurcated social media presence.
Emotion and Sentiment
Overall, Trump's personal account displays more emotion in general compared to the official POTUS account. However, levels of each type of emotion measured for each account via the NRC Emotion Lexicon appear to be somewhat correlated. Trust has the highest score for both accounts, followed by anticipation and then fear.
Additionally, Trump's personal account displays a greater amount of both positive and negative sentiment; although, when positive vs. negative sentiment is compared to obtain sentiment valence, it becomes clear that the official POTUS account is on balance more positive than Trump's personal account.
However, a Welch two-sample t-test reveals that the difference in mean sentiment valence between accounts falls short of conventional standards for statistical significance.
Topical Prevalence
In an effort to make quantifiable sense out of more than 850 Tweets, following estimation of sentiment valence per Tweet, I then utilized STM analysis to identify topics where sentiment valence and account identity served as metadata for the estimation of topical prevalence and where account identity served as a covariate for topical content. Words in the corpus of Tweets used for the analysis were stemmed. The model was estimated for k = 10 topics for a total of 864 documents (Tweets) with a 2,281 word dictionary. The model converged after 43 expectation-maximization iterations.
The top 10 highest probability words per topic appear below.
Following STM analysis, further regression analysis was performed where expected topic proportions served as the independent variable and sentiment valence and account identity served as covariates. The modeled effects of each covariate on topical prevalence are shown below.
Topic 1
Model estimation for a topic comprised of the following highly correlated word stems reveals these sets of words as a unit experience a rise in expected proportions in response to an increase in sentiment valence: great, thank, America, maga, secur, border, use, wonder, work, tune. Taken together, these results suggest that Trump is likely to mention his quintessential hashtag (#MAGA) in conjunction with words related to border and security, as well as work, all within a context of, on balance, greater positive as opposed to negative sentiment. There, moreover, does not appear to be a significant difference in expected topic proportions as a function of whether the Twitter account is Donald Trump's personal account as opposed to the official POTUS account. Some degree of overlap, however, is to be expected in the event of retweets.
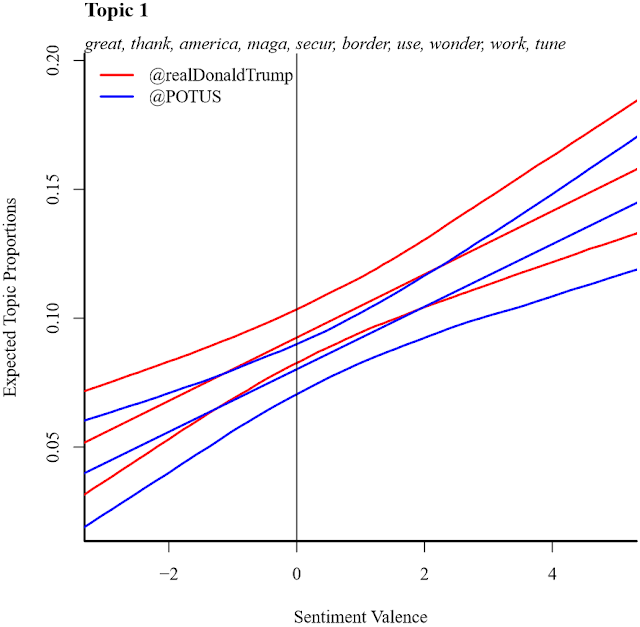
Topic 2
Topic 2, which is comprised of allusions to the Obama Administration, is markedly negative; however, the degree of overlap between expected proportions per account is such that it renders any difference in topic proportions as a function of account identity statistically insignificant from zero.
Topic 3
Topic 3, like Topic 2, is also likely to show up in greater proportions as sentiment valence becomes ever more negative. This would be expected for a topic that appears to focus on subjects typically associated with President Trump's ire: "Obamacare," Russia, allusions to fake news, #repealandreplace, and immigration.
Topic 4
Topic 4 focuses generally on Fox News stories, as well as on mentions of Dan Scavino, who played a role in directing Trump's social media during his campaign and later became one of Trump's senior advisors as President. Expected proportions for this topic increase with negative sentiment, and appear in comparable proportions as a function of account identity.
Topic 5
Topic 5 is the first topic discussed thus far that appears to be significantly associated with one account as opposed to the other. Offers of congratulations, announcements, mentions of women, the need to listen, and #americanspirit are highly correlated and appear to be positively associated with the official POTUS account and have higher estimated proportions in response to an increase in sentiment valence.
Topic 6
Topic 6 appears in near equal proportions per Trump's private Twitter account and the official POTUS account. The topic, which touches on subjects like the White House, making the country better, and words like join and amazing, is expected to experience a significant increase in topical proportions in response to an increase in sentiment valence.
Topic 7
Topic 7 is significantly and positively associated with Trump's personal account. Consisting of highly correlated mentions of the media, news, both major U.S. political parties, election, and disaster, expected topic proportions decline significantly at higher levels of positive vs. negative sentiment.
Topic 8
Highly correlated mentions of #whitehouse, and #potus, and allusions to democrats, Presidential addresses, etc. are most associated with the official POTUS account. Expected topic proportions decline slightly in response to a positive change in sentiment valence, however the degree of change is not substantial.
Topic 9
Topic 9 appears to be an especially positive topic, associated with mentions of #flotus (First Lady of the United States), happiness, tremendous help, and so on. A great deal of overlap between Twitter accounts, however, appears present, which, as mentioned before, may suggest a high degree of retweets between accounts.
Topic 10
Little difference in topic proportions exists between Trump's personal Twitter account and the official POTUS account for Topic 10, a topic that largely centers on signing executive orders. The topic itself experiences an increase in expected proportions in response to greater levels of positive sentiment.
Conclusion
Few people actually question whether @realDonaldTrump or @POTUS represents the true voice of the President -- most people generally suspect the former is the most likely candidate. Even so, Trump appears to be using both accounts in a somewhat strategic manner. His personal account serves as the President's "private face," containing greater emotion, negative sentiment, and more frequent mentions of topics related to media, "Obamacare," and immigration, among others. Meanwhile, the official POTUS account functions as the "public face" of the Presidency, dealing with announcements and other official White House business and so forth. These findings seem hardly shocking, but most often the proposed difference between the role each account plays for the President is based largely on subjective impressions of each account. It is, therefore, both informative and important that quantitative analysis bears out a similar conclusion. However, in the future, accounting for retweets would be helpful in better parsing out significant differences between both Twitter accounts.________________________________________________
See this project in my GitHub repository here.
Comments
Post a Comment